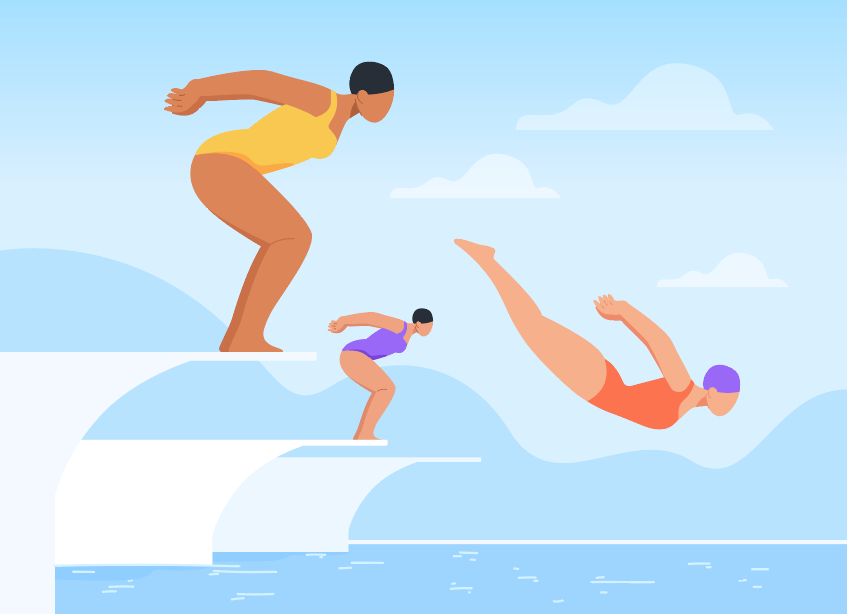
Well, this is the biggie! The final participant has done the final follow-up assessment (i.e., the assessment that happens after they’ve had their therapy), and we now have about a year to dive into the data and analyse it. Because we’re using mixed methods – that’s a combination of qualitative and quantitative data, or to put it simply, words and numbers – there’s quite a lot of work to do and it won’t be quick. First we have to analyse the quantitative data, then we have to analyse the qualitative data (although in reality we’re doing both at the same time), and then we have to bring them together (a “synthesis”) to answer each objective of the study. And that just takes time!
If we’d asked all the study participants to give us numbers (quantitative data) in answer to our questions (for instance: How acceptable was your therapy to you, on a scale of 1-10?) we’d be laughing now: number-based answers to questions like that are very quick to analyse. And we did ask some number-based questions. But we were also asking qualitative questions, i.e., those that needed words to answer them (for instance: In what ways do you feel your therapy made a difference to you?). We asked questions in two different ways: first, using a questionnaire (all participants filled that in), and second, in an interview lasting around half an hour (for 16 of the participants).
Analysing quantitative, analysing qualitative
To analyse the quantitative data, we mostly used “count data” (simply counting numbers and expressing them as numbers and percentages). Most effectiveness trials use much more complex statistical methods, such as some flavour of analysis of variance (ANOVA) that detects differences over time, or linear regression that allows you to predict results based on values, while controlling for additional values. But we’re only looking at feasibility – and we didn’t need complicated methods to count how many people we actually enrolled, or how many people were retained by the end of the trial.
For the qualitative data, we used an analysis method called reflexive thematic analysis (RTA), developed by two researchers called Virginia Braun (University of Auckland) and Victoria Clarke (University of the West of England). There are a lot of academic papers on RTA (I recommend Reflecting on reflexive thematic analysis) but the really rich stuff is on Braun and Clarke’s website. I also recommend David Byrne’s excellent worked example article from 2022, in which Byrne holds your hand and walks you through it if you haven’t done it before. RTA is a six-stage process that takes the researcher through familiarisation, coding, theme generation, development and final definition, and writing up; it’s not uncommon to go backwards to revisit a previous stage before going forwards (Braun and Clarke call that an “iterative” process). If RTA doesn’t sound quick, well, you’re right – it isn’t!
There are many different methods of analysing qualitative data, and RTA wasn’t the only candidate for Santé-AF. But RTA was a good choice because I’m not only the researcher but also a practising, qualified acupuncturist, and that means I probably have some bias towards acupuncture, even if I’m not aware of it. And there are also lots of other ways in which I unconsciously “interpret” all kinds of things according to my own beliefs, values, culture, upbringing, and so on – just like everyone does, but in research this is a really important thing to be aware of. I used a reflexive approach to analysis (although in truth, it wasn’t just in the data analysis – I was using this technique from the very beginning of study design) that meant I kept an awareness of my own “positionality” front and centre. I wrote a “positionality statement” declaring the ways in which my identity, beliefs and values might shape the ways I interpret the qualitative data; I kept this in mind throughout the data analysis, and I’m making a lot of entries in a research journal in which I get to reflect on how my unconscious biases might be at work. Those biases definitely are at work – not just my professional identity, but also my beliefs and values in other spheres, and when I come up against my own assumptions it’s often not comfortable. I can’t eliminate those biases (they’re an essential part of human experience, for me just as for the trial participants) but I am declaring them to make them transparent, and I am continuing to reflect, and often return to the data to re-analyse, to make sure that my biases aren’t shaping the data in a way that distorts it away from (what I believe are) the participants’ intentions. Also, very helpfully, I’m able to call on the study practitioners to query my interpretations or just put the data in front of them to see whether they have a different point of view about it. It’s always helpful to have another pair of eyes, and I’m enduringly grateful to them for their help!
Synthesising the data
One of the really useful things about using mixed methods, especially in a feasibility study, is the synthesis, or the stage where you bring together the qualitative and the quantitative data to answer a question. At that point, the qualitative data can add richness and depth to the numbers, and help the researcher to understand why something has turned out the way it has. So, if you like, the numbers tell you what and the words can tell you why; and bringing those two things together provides a powerful way of understanding what, and how, might need to change in a future trial to make it work better.
A really good example of this would be an exploratory analysis (i.e., one that wasn’t planned, but was of interest) that I carried out to look at the acceptability of the study’s assessments. Some of the participants ended up doing assessments that were two-plus hours long, and I wondered whether that made their assessments less acceptable to them. I fed some numbers into the Statistical Package for Social Sciences (SPSS), a software package for analysing quantitative data, using a method known as a Somers’ d test, to find out whether there was any association between the length of assessments (measured in minutes and divided into half-hour durations) and the overall acceptability of assessments (measured on a 1–7 scale, where higher numbers = greater acceptability). The test showed that there was no association between assessment length and assessment acceptability; in fact, some of the participants giving the highest ratings for assessment acceptability had the longest assessments! That was completely the opposite of what I’d expected. So I brought those numbers together with the qualitative data that participants had written in the questionnaires, or spoken in their interviews, to understand why some participants had found longer assessments more acceptable than others who had shorter assessments. Straight away I found a theme, illustrated by these quotes:
I genuinely enjoyed doing it. Because it gave me this opportunity to articulate a few of the things that have been going on with me that normally are never explored.
Participant 4/910
Obviously these things can be therapeutic and being able to talk about them, so that’s… definitely a positive thing.
Participant 2/515
[The assessments] haven’t been inconvenient… and you know, a pleasant experience just talking about what I’ve experienced and what you’re trying to get out of us.
Participant 3/641
All these participants had had longer assessments; 2/515 in particular had the longest assessments of all the participants, clocking in at an average two and a half hours. All these quotes suggested that the participants had gained some enjoyment, pleasure or even therapeutic value from their assessments, and as a result they hadn’t minded how long the assessment took. So, while we did make some recommendations about shortening the assessments in a future trial, we also didn’t focus those recommendations on the parts of the assessments where participants got to talk about their experiences and feelings, as this was clearly “a positive thing” in the words of Participant 2/515.
So you can see there’s a lot of data analysis to get through. It’s enjoyable, and very absorbing – and it’s taking a very long time!